Kernel methods have been very useful to solve nonlinear identification problems. The main drawback of these methods resides in the fact that the number of elements of the kernel development, i.e., the size of the dictionary, increases with the number of input data, making the solution not suitable for online problems especially time series applications, adaptive filtering and online identification.
Our main contributions are described in the following, from newer to older, with some selected publications.
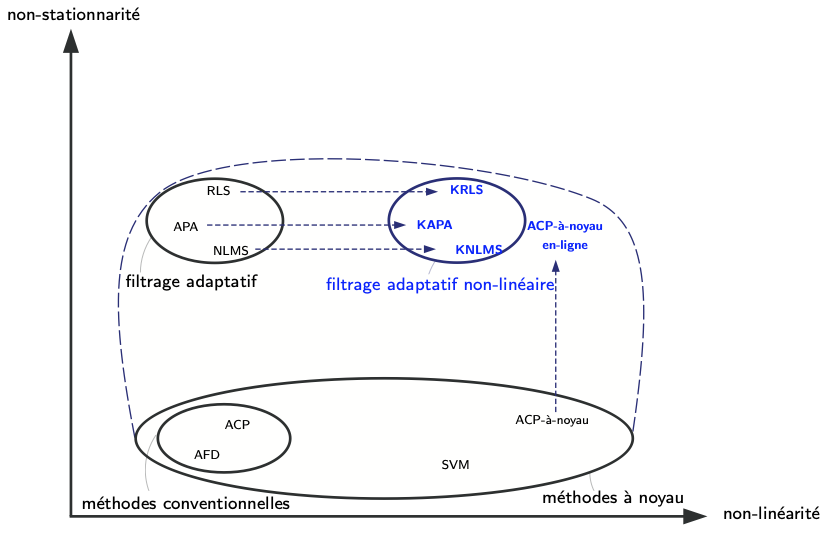
Framework for Online Sparsification Criteria (2015-2016)
We provide a framework that englobes the state-of-the-art online sparsification criteria, such as the distance, linear approximation, coherence, Babel (or cumulative coherence) and entropy. Within this framework, we derive many theoretical results, including bounds on the approximation errors and eigenvalues associated to the dictionary.
P. Honeine
Approximation errors of online sparsification criteria
IEEE Trans. on Signal Processing, 63 (17): 4700-4709, Sept. 2015
P. Honeine
Analyzing sparse dictionaries for online learning with kernels
IEEE Trans. on Signal Processing, 63 (23), 6343-6353, Dec. 2015
Dictionary online adaptation (2013-2015)
See page Online Dictionary Adaptation (Sparse Learning) for details…
C. Saidé, R. Lengellé, P. Honeine, C. Richard, and R. Achkar
Nonlinear Adaptive Filtering using Kernel-based Algorithms with Dictionary Adaptation
International Journal of Adaptive Control and Signal Processing, 29 (11), 1391-1410, Nov. 2015
C. Saidé, R. Lengellé, P. Honeine, and R. Achkar
Online kernel adaptive algorithms with dictionary adaptation for MIMO models
IEEE Signal Processing Letters, 20 (5), 535-538, May 2013
Unsupervised Nonlinear Online Learning (2012)
This is the first time that online sparsification, initially derived for nonlinear adaptive filtering, is extended to unsupervised learning.
P. Honeine
Online kernel principal component analysis: a reduced-order model
IEEE Transactions on Pattern Analysis and Machine Intelligence, 34 (9), 1814-1826, Sep. 2012
Online Detection with Adaptive One-class Learning (2011-2014)
See page …. for details…
Adaptive Learning for Wireless Sensor Networks (2008-2011)
See page Collaborative Learning in (Wireless) Sensor Networks for details…
Adaptive Learning with Nonlinear Adaptive Algorithms (2005-2009)
Here, we proposed the coherence criterion for online sparsification, and developed the corresponding algorithms (Kernel Recursive Least Squares, Kernel (Normalized) Least Mean Squares and Kernel Affine Projection)
C. Richard, J. C. M. Bermudez, and P. Honeine
Online prediction of time series data with kernels
IEEE Trans. on Signal Processing
57(3):1058-1067, 2009
P. Honeine, C. Richard, J. C. M. Bermudez, and H. Snoussi
Distributed prediction of time series data with kernels and adaptive filtering techniques in sensor networks