Nonlinear adaptive filtering has been extensively studied in the literature, using for example Volterra filters or Neural Networks. Recently, kernel methods have been offering an interesting alternative since they provide a simple extension of linear algorithms to the nonlinear case. The main drawback of online system identification with kernel methods is that the filter complexity increases with time, a limitation resulting from the representer theorem which states that all past input vectors (i.e., training samples) are required. To overcome this drawback, a particular subset of these input vectors (called dictionary) must be selected to ensure complexity control and good performance. See page Online Learning and Nonlinear Adaptive Filtering for more details.
Prior to our work, all authors considered that, after being introduced into the dictionary, elements stay unchanged even if, due to nonstationarity, they become useless to predict the system output. Our work presents an adaptation scheme of dictionary elements, which are considered in our framework as adjustable model parameters. To this end, we derive several algorithms, such as a gradient-based method under collinearity constraints. By adapting the dictionary elements, we ensure a better tracking performance, as confirmed by experiments where complexity reduction and a decrease of the instantaneous quadratic error are observed.
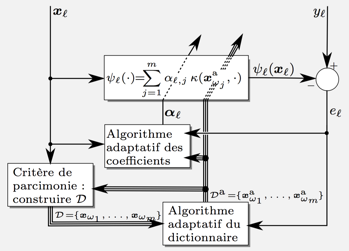
Selected Papers
C. Saidé, R. Lengellé, P. Honeine, C. Richard, and R. Achkar
Nonlinear Adaptive Filtering using Kernel-based Algorithms with Dictionary Adaptation
International Journal of Adaptive Control and Signal Processing, 29 (11), 1391-1410, Nov. 2015
C. Saidé, R. Lengellé, P. Honeine, and R. Achkar
Online kernel adaptive algorithms with dictionary adaptation for MIMO models
IEEE Signal Processing Letters, 20 (5), 535-538, May 2013
C. Saidé, R. Lengellé, P. Honeine, C. Richard, and R. Achkar
Dictionary Adaptation for Online Prediction of Time Series Data with Kernels
Proc. IEEE workshop on Statistical Signal Processing (SSP), Ann Arbor, Michigan, USA, pp. 604-607, 5-8 Aug. 2012
C. Saidé, P. Honeine, R. Lengellé, C. Richard, and R. Achkar
Adaptation en ligne d’un dictionnaire pour les méthodes à noyaux
Actes du 24-ème Colloque GRETSI sur le Traitement du Signal et des Images, Brest, France, Sept. 2013